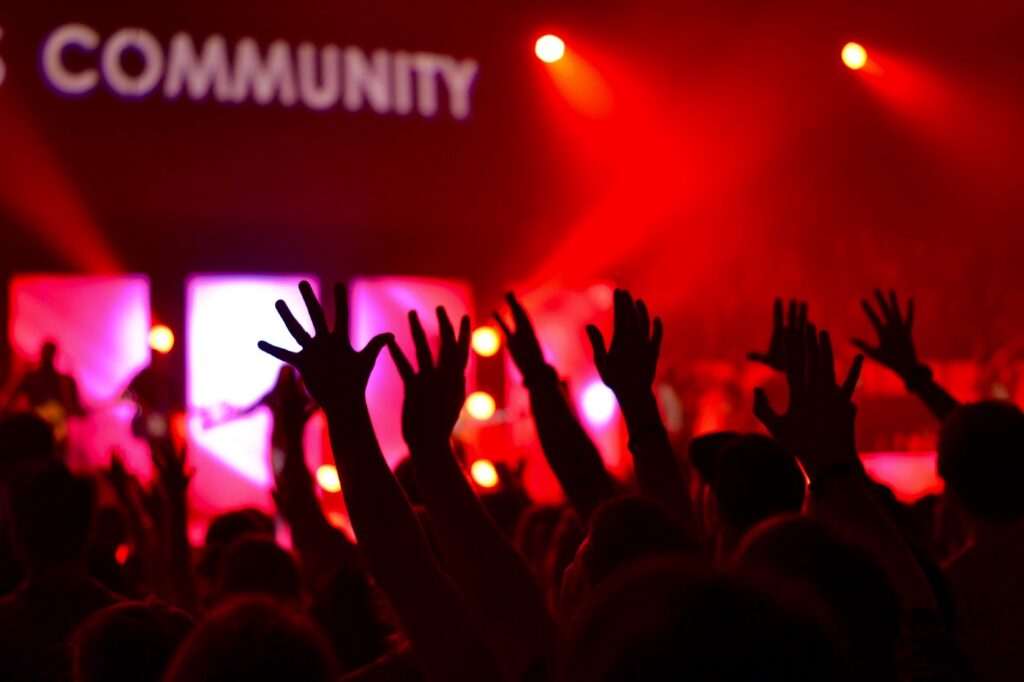
Many are familiar with the phrase it takes a village to raise a child, but where did it come from and what does it mean? It is widely believed to be an African proverb that may have biblical origins reflecting African cultures’ emphasis on family and community. However, in America, it is commonly used in its shortened form; it takes a village to describe a situation that requires the help of many to achieve a common objective.
In the world of cybersecurity, it has long been known that information sharing is the best way to help the community defend against attacks, especially considering those that lack resources or expertise to go it alone. The concept has spawned entire industries around threat intelligence, the creation of information sharing organizations, and government regulation. Yet, as a whole, we continue to struggle with sharing, either due to privacy concerns, fear of retribution, lack of trust in the data, and many other reasons.
Why is it so hard?
Well, for that answer, we first must examine what information is shared. The most fundamental and familiar piece of information shared is an indicator of compromise (IOC). IOCs are typically hashes or file names of malware, IP addresses of C2 servers or other adversary infrastructure, etc. The problem with IOCs is they are ephemeral. A hacker merely needs to spin up new infrastructure or tweak their malware to change its hash to get around existing detections. Additionally, attacks have increased in complexity resulting in massive increases in the volume of IOC data. The data needs to be managed, investigated, and validated, and it is a significant undertaking. By the time the task is complete, the data is often outdated and ignored or, conversely, blindly ingested into one’s environment without concern for the potential massive increase in false positives. The increase creates an enormous burden on operators and analysts and contributes to alert fatigue.
Next, we must look at how information is shared. The most formal groups usually have a repository where participants can, and in some cases, are required to upload data to a system where others can access the shared data. These often lack the context needed to make any accurate decisions around the applicability of those IOCs to one’s organization. Conversely, there is sharing among peer groups using informal channels or private groups. The information shared in these is often high quality and applicable to the recipients but does not have a wide enough distribution to impact security as a whole. Lastly, there are communities centered around open source tools and repositories, which are an excellent resource for those who cannot afford data subscriptions but struggle with curating the data, context, and applicability to the user, and at times quality of information.
Finally, we must look inward. There are real concerns when it comes to information sharing for companies. Attribution of information around threat sharing can be evidence (or assumption) of an undisclosed attack or even a breach. This can adversely affect everything from an investigation into a lack of reporting to a dip in stock price or a loss of customers. Thus, the incentive for sharing beyond regulatory requirements is limited and often restricted by policy.
Any conversation about threat data and information sharing would be remiss if it did not mention the commercial threat intelligence market. Although it is not technically information sharing, it is comprised of information exchange. Threat intelligence is a must to operate as a threat-informed security program, but the market offerings have huge inconsistencies in data quality, scope, focus, and usability. Some feeds focus on verbose reports requiring consumption and understanding by the reader, while other sources are strictly data feeds of IOCs with quality, coverage, and timeliness varying wildly from vendor to vendor. Additionally, there are open source and free threat intelligence data sources that range from high-quality and trusted to those with limited resources for maintenance and collection. The reality is the perfect threat intelligence offering is often a blend of a few sources and vendors to meet the collection requirements of the customer if they have the budget and staff to manage the incoming data. And the marketing in this space makes it challenging to understand who has the best data for the specific customer requirements. The result is either an overspend on data with a lack of ROI, an underspend on data and reliance on people to research and cull from sources such as Twitter, or threat data is forgone altogether for those lacking either budget or staff.
Again the takeaway is that the vast majority of the shared data are ephemeral IOCs with a limited shelf life. The challenge lies with security teams constantly plugging holes, refreshing IOC-based detections, and organically researching information when triaging alerts, all the while hoping that the IOC coverage is complete and current enough at any given point in time.
How can we make this better?
Start with the data
First and foremost, we need to seek data that is far less ephemeral for our defenses. Not that IOCs do not have a place in security, but leveraging the process of attack in the form of behavioral detections allows teams to cover the easy permutations of things like changing a filename, IP address, or domain without having to update their detection logic. In the form of a behavioral analytic describing the processes and artifacts invoked during an attack, this data is significantly more robust than alerting on a specific IOC alone. Additionally, IOCs are typically associated with a specific threat or actor, while behaviors can cover multiple threats with a single analytic, significantly reducing the burden on detection engineering.
Focus on data quality
What good is having data if we are not confident it is both accurate and current? We can effectively automate what constitutes a “good” analytic using both data science coupled with true positive attack data. We can test the coverage and robustness of a given analytic while also validating the detection logic using attack data. Being able to emulate a true positive attack against a machine running an analytic detection provides the assurance that the detection does perform as expected against the threat. By providing this data coupled together, defenders can quickly validate and ensure their detections in their environment will function when exposed to the threat’s behavior. This data can further be used to ensure detections remain effective as part of regular, automated controls testing. The key is transparently validating all analytic data — shared or organically created — against key features and true positive attacks so as to certify it as high-quality, robust, and trusted.
Ensure data usability
When starting with high-quality and trusted data, the data can be immediately ingested and used — that is, if it is in a format that matches the security tools in use. Unlike IOCs that are inherently tool-agnostic and are a relatively easily ingested piece of information, behavioral analytics, unfortunately, are not. So the shared analytic must be easily and automatically converted across common languages to support a wide breadth of security tools. There are some open-source tools that support this capability at a generic level, but the analytic still must be tuned to one’s environment to minimize false positives. Ideally, the data can be automatically updated on ingestion to be able to handle parameters to customize an analytic to account for non-standard installations of security tools or to filter out known-good security operations and minimize the reliance on human operator expertise.
Understand the threat landscape in your context
Using a standard framework, such as MITRE ATT&CK, as a baseline for scoping the threat landscape is a start. Applying an overlay of your monitoring and data sources provides a data coverage map showing organizational blind spots. And lastly, overlaying validated analytic deployments will provide a view of detection gaps. Together an organization can deeply understand their evolving risk in an ever-changing threat landscape.
Though this is easier said than done and comes back to quality data. For an organization to be effective, each attack and analytic must be properly mapped back to its framework of choice. Associating each analytic with the context around the specific threats it detects and related tools, techniques, and procedures (TTP) as tags or labels in the dataset enables defenders to find, analyze, and deploy the information quickly. Using a standard framework as a baseline for labeling creates a common, accepted, and generally understood vernacular that ties the data back to the known threat landscape. Tracking deployment of the labeled analytic data to an organization’s environment and validating those detections enables organizations to understand their coverage across the threat landscape, identify gaps, understand risk, and develop budgets and plans.
Collaborate on offensive and defensive security
Traditionally, the offensive (red) and the defensive (blue) teams have competing missions and operate separately with little collaboration. This leaves detection engineering as a lagging process. Only with practical and continuous cooperation between those creating and contextualizing attacks and threats (threat analysts, security researchers, penetration testers, and red teamers) and those defining, deploying, and monitoring detections (hunters, security operators, and blue teamers), can we streamline these processes and reduce the time to detection. A collaborative and iterative approach of defining attacks and detections as a single unit results in the most robust, validated, and complete attack possible. This data can be immediately shared, understood, trusted, and consumed since the detection analytic has built-in transparency of the true positive attack data.
Nurture the community
Community plays a huge part in being able to stay ahead of adversaries. As threats evolve, exploits uncovered, vulnerabilities announced, or if our defenses miss, it is vital that the attack information is shared out to the community for a rapid and wide response. The nature of attack information is such that some are less inclined to share for fear of attribution, so the community must be provided with a way to disseminate the data in an anonymous way, modify the shared data as more information is uncovered, create or iterate on detection analytics, and certify the data is valid and trustworthy. The data must be easy to find, link, consume, use, and monitor. And the community must be supported with modern tool interfaces to help with lowering the skill required to create and access analytic data and drastically remove barriers to consuming the data.
As the community contributes attack and analytic data, the same community should refine and validate the data without losing data integrity and quality. The community should be able to work together and share information without fear of retribution and have confidence in data quality. Ultimately, the community needs a place to crowdsource the best analytics and attack data while providing the transparency and trust required to consume the data directly as enterprise-grade.
It takes a village…to stop hackers
At SnapAttack, we believe cybersecurity is only as strong as the weakest link. Only by working together can we collectively gather enough information to prevent attacks and stay on top of new ones. By bridging the actions of red teams and blue teams, we can create a collaborative purple team at community scale. SnapAttack is created to lower the barrier to entry such that anyone can contribute, collaborate, use, hunt, simulate, deploy, emulate, and validate behavioral analytics in their network using an intuitive graphical user interface, machine-based scoring, and integrations. Ultimately, our platform is centered around a library of vendor-neutral behavioral analytics and threat data married together in such a way that analytics are validated against true-positive attacks for confidence in functionality and visibility into threat coverage.
Enjoy learning about innovations in cybersecurity and proactive security? Find out more or get in touch.